Multimodal language models (LMMs) are a transformative technology that blends natural language processing with visual data interpretation. Their applications extend to multilingual virtual assistants, cross-cultural information retrieval, and content understanding. By combining linguistic comprehension and image analysis, LMMs promise enhanced accessibility to digital tools, especially in linguistically diverse and visually rich contexts. However, their effectiveness hinges on their ability to adapt to cultural and linguistic nuances, a challenging task given the diversity of global languages and traditions.
One of the critical challenges in this field is the need for more performance of LMMs in low-resource languages and culturally specific contexts. While many models excel in high-resource languages like English and Mandarin, they falter with languages such as Amharic or Sinhala, which have limited training data. Furthermore, cultural knowledge is often underrepresented, with existing models needing help interpreting traditions, rituals, or domain-specific information. These limitations reduce the inclusivity and utility of LMMs for global populations.
Benchmarks for evaluating LMMs have historically needed to be improved. CulturalVQA and Henna benchmarks, for instance, cover a limited number of languages and cultural domains. CulturalVQA focuses primarily on English and culturally specific content, while Henna addresses cultural aspects in Arabic across 11 countries but needs more breadth in domain and language diversity. Existing datasets are often skewed towards high-resource languages and single-question formats, incompletely evaluating a model’s cultural and linguistic abilities.
Researchers from the University of Central Florida, Mohamed bin Zayed University of AI, Amazon, Aalto University, Australian National University, and Linköping University introduced the All Languages Matter Benchmark (ALM-bench) to address these shortcomings. This extensive framework evaluates LMMs across 100 languages from 73 countries, including high- and low-resource languages. The benchmark encompasses 24 scripts and 19 cultural and generic domains, ensuring comprehensive linguistic and cultural representation.
The methodology behind ALM-bench is rigorous and data-driven. It includes over 22,763 manually verified question-answer pairs, categorized into 6,000 general VQA pairs and 16,763 culturally specific ones. Question formats range from multiple-choice to true/false and visual question answering (VQA), ensuring a thorough evaluation of multimodal reasoning. The data were collected using GPT-4o translations, later refined by native language experts, with more than 800 hours dedicated to annotation. Care was taken to include images and cultural artifacts representing 13 distinct domains, such as architecture, music, festivals, and notable key figures, reflecting cultural depth and diversity.
Evaluation results revealed significant insights into the performance of 16 state-of-the-art LMMs. Proprietary models like GPT-4o and Gemini-1.5-Pro outperformed open-source models, achieving 78.8% and 74.3% accuracy, respectively. While closed-source models excelled in high-resource languages, they showed a steep performance drop for low-resource ones. For example, GPT-4o’s accuracy fell from 88.4% for English to 50.8% for Amharic. Open-source models like GLM-4V-9B performed better than others in their category but remained less effective, with an overall accuracy of 51.9%. The benchmark also highlighted disparities across cultural domains, with the best results in education (83.7%) and heritage (83.5%) and weaker performance in interpreting customs and notable key figures.
This research provides several critical takeaways that underscore the significance of ALM-bench in advancing LMM technology:
- Cultural Inclusivity: ALM-bench sets a new standard by including 100 languages and 73 countries, making it the most comprehensive benchmark for LMM evaluation.
- Robust Evaluation: The benchmark tests models’ ability to reason about complex linguistic and cultural contexts using diverse question formats and domains.
- Performance Gaps: The study identified a stark contrast between high-resource and low-resource languages, urging more inclusive model training.
- Proprietary vs. Open Source: Closed-source models consistently outperformed open-source counterparts, showcasing the importance of proprietary innovations.
- Model Limitations: Even the best models struggled with nuanced cultural reasoning, emphasizing the need for improved datasets and training methodologies.

In conclusion, the ALM-bench research sheds light on the limitations of multimodal language models while offering a groundbreaking framework for improvement. By encompassing 22,763 diverse questions across 19 domains and 100 languages, the benchmark fills a critical gap in evaluating linguistic and cultural inclusivity. It highlights the need for innovation to address disparities in performance between high- and low-resource languages, ensuring these technologies are more inclusive and effective for a global audience. This work paves the way for future developments in AI to embrace and reflect the rich tapestry of global languages and cultures.
Check out the Paper and Project. All credit for this research goes to the researchers of this project. Also, don’t forget to follow us on Twitter and join our Telegram Channel and LinkedIn Group. If you like our work, you will love our newsletter.. Don’t Forget to join our 55k+ ML SubReddit.
🎙️ 🚨 ‘Evaluation of Large Language Model Vulnerabilities: A Comparative Analysis of Red Teaming Techniques’ Read the Full Report (Promoted)
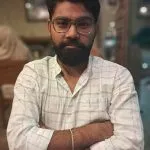
Nikhil is an intern consultant at Marktechpost. He is pursuing an integrated dual degree in Materials at the Indian Institute of Technology, Kharagpur. Nikhil is an AI/ML enthusiast who is always researching applications in fields like biomaterials and biomedical science. With a strong background in Material Science, he is exploring new advancements and creating opportunities to contribute.
Credit: Source link