Generative agents are computational models replicating human behavior and attitudes across diverse contexts. These models aim to simulate individual responses to various stimuli, making them invaluable tools for exploring human interactions and testing hypotheses in sociology, psychology, and political science. By integrating artificial intelligence, these agents offer novel opportunities to enhance understanding of social phenomena and refine policy interventions through controlled, scalable simulations.
The challenge this research addresses lies in the limitations of traditional models for simulating human behavior. Existing approaches often rely on static or demographic-based attributes, which oversimplify the complexity of human decision-making and fail to account for individual variations. This lack of flexibility restricts their application in studies requiring nuanced representations of human attitudes and behaviors, creating demand for more dynamic and precise systems.
Historically, simulations of human behavior have been conducted through agent-based models and demographic profiling, which rely on predefined attributes and are constrained by interpretability. Recent advancements in artificial intelligence, particularly large language models, have demonstrated the ability to generalize human behavior across contexts. However, these systems face criticism for propagating stereotypes and failing to represent individual diversity accurately. Researchers have sought to overcome these challenges by integrating richer datasets and adaptive architectures into their models.
The research team from Stanford University, in collaboration with Google DeepMind, Northwestern University, and the University of Washington, developed a novel generative agent architecture. The system incorporates interview-based datasets, capturing in-depth individual data through semi-structured qualitative interviews. The participants included a stratified sample of 1,052 individuals from the United States, ensuring representation across age, race, gender, and political ideologies. The interviews were conducted using a custom AI interviewer, which dynamically adapted questions to the participants’ responses. By integrating this detailed data with a large language model, the researchers created simulations capable of accurately predicting individual attitudes and behaviors while reducing biases commonly associated with demographic-based approaches.
The architecture utilizes participants’ comprehensive interview transcripts as the foundation for the simulations. When prompted, the agents draw from the full interview data to respond contextually. To evaluate their effectiveness, the researchers benchmarked the agents against responses to the General Social Survey (GSS), Big Five personality traits inventory, and several economic games. The agents also participated in experimental replications of well-known behavioral studies. The research team ensured rigorous evaluation metrics by normalizing accuracy against participants’ consistency in retaking the same surveys two weeks later. Memory mechanisms further enhanced the agents’ ability to simulate multi-step interactions, allowing them to adapt and learn from prior responses.
The results of the study demonstrated significant improvements over existing methods. Generative agents achieved a normalized accuracy of 0.85 on the General Social Survey, reflecting an 85% match to participants’ responses. By comparison, demographic-based agents scored 0.71, and persona-based agents scored 0.70. In predicting the Big Five personality traits, the agents recorded a correlation of 0.80, outperforming baseline methods by a substantial margin. Economic game simulations also showed high accuracy, with normalized correlations of 0.66 for decision-making tasks. These agents consistently outperformed benchmarks, including when interview data was reduced by up to 80%, underscoring the robustness of the architecture.
Moreover, the research highlighted a significant reduction in bias across demographic subgroups. For political ideology, the performance disparity between favored and less favored groups dropped from 12.35% for demographic-based agents to 7.85% for interview-based agents. Similarly, the disparity decreased significantly in personality and economic game predictions, indicating the system’s ability to produce fairer and more inclusive simulations. The results of experimental replications further reinforced the agents’ predictive accuracy, as they replicated findings from four out of five behavioral studies with strong correlations to human participants’ responses.
In conclusion, this study presents a breakthrough in behavioral simulations by leveraging detailed qualitative data and advanced AI architectures. The generative agents developed by the Stanford University and Google DeepMind research team address longstanding limitations in traditional models, offering a scalable and ethically grounded solution for simulating human behavior. This advancement improves predictive accuracy and sets the stage for future social science and policy development applications. By reducing biases and incorporating rich datasets, the research underscores the potential of AI in creating tools that reflect the complexity of human interactions.
Check out the Paper. All credit for this research goes to the researchers of this project. Also, don’t forget to follow us on Twitter and join our Telegram Channel and LinkedIn Group. If you like our work, you will love our newsletter.. Don’t Forget to join our 55k+ ML SubReddit.
[FREE AI VIRTUAL CONFERENCE] SmallCon: Free Virtual GenAI Conference ft. Meta, Mistral, Salesforce, Harvey AI & more. Join us on Dec 11th for this free virtual event to learn what it takes to build big with small models from AI trailblazers like Meta, Mistral AI, Salesforce, Harvey AI, Upstage, Nubank, Nvidia, Hugging Face, and more.
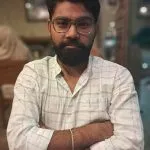
Nikhil is an intern consultant at Marktechpost. He is pursuing an integrated dual degree in Materials at the Indian Institute of Technology, Kharagpur. Nikhil is an AI/ML enthusiast who is always researching applications in fields like biomaterials and biomedical science. With a strong background in Material Science, he is exploring new advancements and creating opportunities to contribute.
Credit: Source link