Machine learning has achieved remarkable advancements, particularly in generative models like diffusion models. These models are designed to handle high-dimensional data, including images and audio. Their applications span various domains, such as art creation and medical imaging, showcasing their versatility. The primary focus has been on enhancing these models to better align with human preferences, ensuring that their outputs are useful and safe for broader applications.
Despite significant progress, current generative models often need help aligning perfectly with human preferences. This misalignment can lead to either useless or potentially harmful outputs. The critical issue is to fine-tune these models to consistently produce desirable and safe outputs without compromising their generative abilities.
Existing research includes reinforcement learning techniques and preference optimization strategies, such as Diffusion-DPO and SFT. Methods like Proximal Policy Optimization (PPO) and models like Stable Diffusion XL (SDXL) have been employed. Furthermore, frameworks such as Kahneman-Tversky Optimization (KTO) have been adapted for text-to-image diffusion models. While these approaches improve alignment with human preferences, they often fail to handle diverse stylistic discrepancies and efficiently manage memory and computational resources.
Researchers from the Korea Advanced Institute of Science and Technology (KAIST), Korea University, and Hugging Face have introduced a novel method called Maximizing Alignment Preference Optimization (MaPO). This method aims to fine-tune diffusion models more effectively by integrating preference data directly into the training process. The research team conducted extensive experiments to validate their approach, ensuring it surpasses existing methods in terms of alignment and efficiency.
MaPO enhances diffusion models by incorporating a preference dataset during training. This dataset includes various human preferences the model must align with, such as safety and stylistic choices. The method involves a unique loss function that prioritizes preferred outcomes while penalizing less desirable ones. This fine-tuning process ensures the model generates outputs that closely align with human expectations, making it a versatile tool across different domains. The methodology employed by MaPO does not rely on any reference model, which differentiates it from traditional methods. By maximizing the likelihood margin between preferred and dispreferred image sets, MaPO learns general stylistic features and preferences without overfitting the training data. This makes the method memory-friendly and efficient, suitable for various applications.
The performance of MaPO has been evaluated on several benchmarks. It demonstrated superior alignment with human preferences, achieving higher scores in safety and stylistic adherence. MaPO scored 6.17 on the Aesthetics benchmark and reduced training time by 14.5%, highlighting its efficiency. Moreover, the method surpassed the base Stable Diffusion XL (SDXL) and other existing methods, proving its effectiveness in generating preferred outputs consistently.
The MaPO method represents a significant advancement in aligning generative models with human preferences. Researchers have developed a more efficient and effective solution by integrating preference data directly into the training process. This method enhances the safety and usefulness of model outputs and sets a new standard for future developments in this field.
Overall, the research underscores the importance of direct preference optimization in generative models. MaPO’s ability to handle reference mismatches and adapt to diverse stylistic preferences makes it a valuable tool for various applications. The study opens new avenues for further exploration in preference optimization, paving the way for more personalized and safe generative models in the future.
Check out the Paper. All credit for this research goes to the researchers of this project. Also, don’t forget to follow us on Twitter.
Join our Telegram Channel and LinkedIn Group.
If you like our work, you will love our newsletter..
Don’t Forget to join our 45k+ ML SubReddit
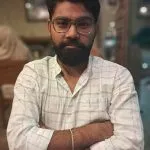
Nikhil is an intern consultant at Marktechpost. He is pursuing an integrated dual degree in Materials at the Indian Institute of Technology, Kharagpur. Nikhil is an AI/ML enthusiast who is always researching applications in fields like biomaterials and biomedical science. With a strong background in Material Science, he is exploring new advancements and creating opportunities to contribute.
Credit: Source link