This article discusses a new release of a multimodal Hunyuan Video world model called ‘HunyuanCustom’. The new paper’s breadth of coverage, combined with several issues in many of the supplied example videos at the project page*, constrains us to more general coverage than usual, and to limited reproduction of the huge amount of video material accompanying this release (since many of the videos require significant re-editing and processing in order to improve the readability of the layout).
Please note additionally that the paper refers to the API-based generative system Kling as ‘Keling’. For clarity, I refer to ‘Kling’ instead throughout.
Tencent is in the process of releasing a new version of its Hunyuan Video model, titled HunyuanCustom. The new release is apparently capable of making Hunyuan LoRA models redundant, by allowing the user to create ‘deepfake’-style video customization through a single image:
Click to play. Prompt: ‘A man is listening to music and cooking snail noodles in the kitchen’. The new method compared to both close-source and open-source methods, including Kling, which is a significant opponent in this space. Source: https://hunyuancustom.github.io/ (warning: CPU/memory-intensive site!)
In the left-most column of the video above, we see the single source image supplied to HunyuanCustom, followed by the new system’s interpretation of the prompt in the second column, next to it. The remaining columns show the results from various proprietary and FOSS systems: Kling; Vidu; Pika; Hailuo; and the Wan-based SkyReels-A2.
In the video below, we see renders of three scenarios essential to this release: respectively, person + object; single-character emulation; and virtual try-on (person + clothes):
Click to play. Three examples edited from the material at the supporting site for Hunyuan Video.
We can notice a few things from these examples, mostly related to the system relying on a single source image, instead of multiple images of the same subject.
In the first clip, the man is essentially still facing the camera. He dips his head down and sideways at not much more than 20-25 degrees of rotation, but, at an inclination in excess of that, the system would really have to start guessing what he looks like in profile. This is hard, probably impossible to gauge accurately from a sole frontal image.
In the second example, we see that the little girl is smiling in the rendered video as she is in the single static source image. Again, with this sole image as reference, the HunyuanCustom would have to make a relatively uninformed guess about what her ‘resting face’ looks like. Additionally, her face does not deviate from camera-facing stance by more than the prior example (‘man eating crisps’).
In the last example, we see that since the source material – the woman and the clothes she is prompted into wearing – are not complete images, the render has cropped the scenario to fit – which is actually rather a good solution to a data issue!
The point is that though the new system can handle multiple images (such as person + crisps, or person + clothes), it does not apparently allow for multiple angles or alternative views of a single character, so that diverse expressions or unusual angles could be accommodated. To this extent, the system may therefore struggle to replace the growing ecosystem of LoRA models that have sprung up around HunyuanVideo since its release last December, since these can help HunyuanVideo to produce consistent characters from any angle and with any facial expression represented in the training dataset (20-60 images is typical).
Wired for Sound
For audio, HunyuanCustom leverages the LatentSync system (notoriously hard for hobbyists to set up and get good results from) for obtaining lip movements that are matched to audio and text that the user supplies:
Features audio. Click to play. Various examples of lip-sync from the HunyuanCustom supplementary site, edited together.
At the time of writing, there are no English-language examples, but these appear to be rather good – the more so if the method of creating them is easily-installable and accessible.
Editing Existing Video
The new system offers what appear to be very impressive results for video-to-video (V2V, or Vid2Vid) editing, wherein a segment of an existing (real) video is masked off and intelligently replaced by a subject given in a single reference image. Below is an example from the supplementary materials site:
Click to play. Only the central object is targeted, but what remains around it also gets altered in a HunyuanCustom vid2vid pass.
As we can see, and as is standard in a vid2vid scenario, the entire video is to some extent altered by the process, though most altered in the targeted region, i.e., the plush toy. Presumably pipelines could be developed to create such transformations under a garbage matte approach that leaves the majority of the video content identical to the original. This is what Adobe Firefly does under the hood, and does quite well – but it is an under-studied process in the FOSS generative scene.
That said, most of the alternative examples provided do a better job of targeting these integrations, as we can see in the assembled compilation below:
Click to play. Diverse examples of interjected content using vid2vid in HunyuanCustom, exhibiting notable respect for the untargeted material.
A New Start?
This initiative is a development of the Hunyuan Video project, not a hard pivot away from that development stream. The project’s enhancements are introduced as discrete architectural insertions rather than sweeping structural changes, aiming to allow the model to maintain identity fidelity across frames without relying on subject-specific fine-tuning, as with LoRA or textual inversion approaches.
To be clear, therefore, HunyuanCustom is not trained from scratch, but rather is a fine-tuning of the December 2024 HunyuanVideo foundation model.
Those who have developed HunyuanVideo LoRAs may wonder if they will still work with this new edition, or whether they will have to reinvent the LoRA wheel yet again if they want more customization capabilities than are built into this new release.
In general, a heavily fine-tuned release of a hyperscale model alters the model weights enough that LoRAs made for the earlier model will not work properly, or at all, with the newly-refined model.
Sometimes, however, a fine-tune’s popularity can challenge its origins: one example of a fine-tune becoming an effective fork, with a dedicated ecosystem and followers of its own, is the Pony Diffusion tuning of Stable Diffusion XL (SDXL). Pony currently has 592,000+ downloads on the ever-changing CivitAI domain, with a vast range of LoRAs that have used Pony (and not SDXL) as the base model, and which require Pony at inference time.
Releasing
The project page for the new paper (which is titled HunyuanCustom: A Multimodal-Driven Architecture for Customized Video Generation) features links to a GitHub site that, as I write, just became functional, and appears to contain all code and necessary weights for local implementation, together with a proposed timeline (where the only important thing yet to come is ComfyUI integration).
At the time of writing, the project’s Hugging Face presence is still a 404. There is, however, an API-based version of where one can apparently demo the system, so long as you can provide a WeChat scan code.
I have rarely seen such an elaborate and extensive usage of such a wide variety of projects in one assembly, as is evident in HunyuanCustom – and presumably some of the licenses would in any case oblige a full release.
Two models are announced at the GitHub page: a 720px1280px version requiring 8)GB of GPU Peak Memory, and a 512px896px version requiring 60GB of GPU Peak Memory.
The repository states ‘The minimum GPU memory required is 24GB for 720px1280px129f but very slow…We recommend using a GPU with 80GB of memory for better generation quality’ – and iterates that the system has only been tested so far on Linux.
The earlier Hunyuan Video model has, since official release, been quantized down to sizes where it can be run on less than 24GB of VRAM, and it seems reasonable to assume that the new model will likewise be adapted into more consumer-friendly forms by the community, and that it will quickly be adapted for use on Windows systems too.
Due to time constraints and the overwhelming amount of information accompanying this release, we can only take a broader, rather than in-depth look at this release. Nonetheless, let’s pop the hood on HunyuanCustom a little.
A Look at the Paper
The data pipeline for HunyuanCustom, apparently compliant with the GDPR framework, incorporates both synthesized and open-source video datasets, including OpenHumanVid, with eight core categories represented: humans, animals, plants, landscapes, vehicles, objects, architecture, and anime.
From the release paper, an overview of the diverse contributing packages in the HunyuanCustom data construction pipeline. Source: https://arxiv.org/pdf/2505.04512
Initial filtering begins with PySceneDetect, which segments videos into single-shot clips. TextBPN-Plus-Plus is then used to remove videos containing excessive on-screen text, subtitles, watermarks, or logos.
To address inconsistencies in resolution and duration, clips are standardized to five seconds in length and resized to 512 or 720 pixels on the short side. Aesthetic filtering is handled using Koala-36M, with a custom threshold of 0.06 applied for the custom dataset curated by the new paper’s researchers.
The subject extraction process combines the Qwen7B Large Language Model (LLM), the YOLO11X object recognition framework, and the popular InsightFace architecture, to identify and validate human identities.
For non-human subjects, QwenVL and Grounded SAM 2 are used to extract relevant bounding boxes, which are discarded if too small.
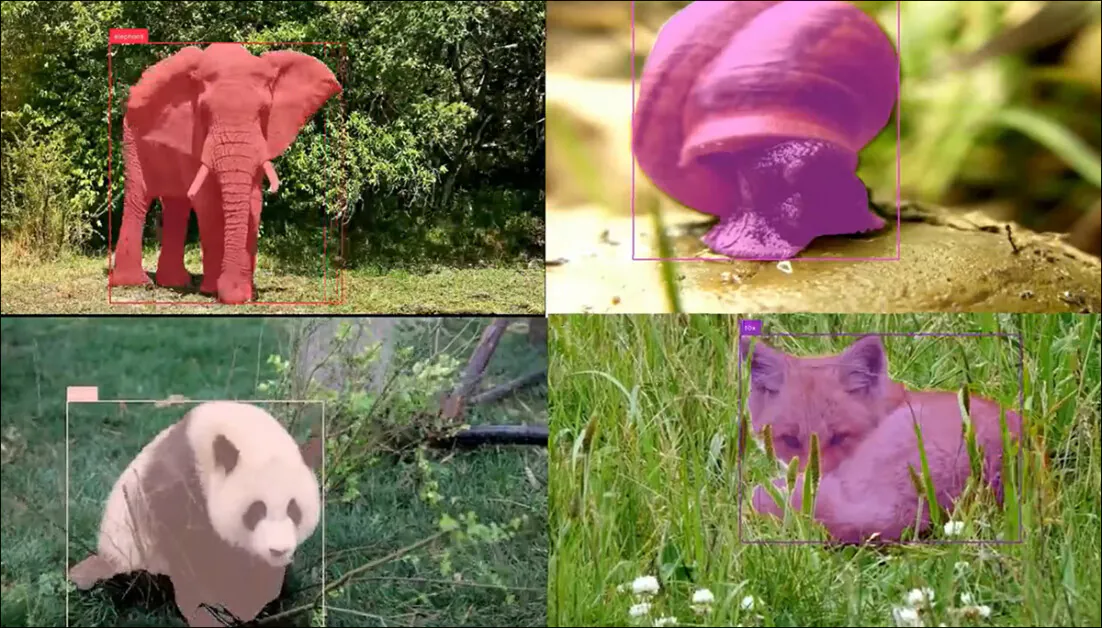
Examples of semantic segmentation with Grounded SAM 2, used in the Hunyuan Control project. Source: https://github.com/IDEA-Research/Grounded-SAM-2
Multi-subject extraction utilizes Florence2 for bounding box annotation, and Grounded SAM 2 for segmentation, followed by clustering and temporal segmentation of training frames.
The processed clips are further enhanced via annotation, using a proprietary structured-labeling system developed by the Hunyuan team, and which furnishes layered metadata such as descriptions and camera motion cues.
Mask augmentation strategies, including conversion to bounding boxes, were applied during training to reduce overfitting and ensure the model adapts to diverse object shapes.
Audio data was synchronized using the aforementioned LatentSync, and clips discarded if synchronization scores fall below a minimum threshold.
The blind image quality assessment framework HyperIQA was used to exclude videos scoring under 40 (on HyperIQA’s bespoke scale). Valid audio tracks were then processed with Whisper to extract features for downstream tasks.
The authors incorporate the LLaVA language assistant model during the annotation phase, and they emphasize the central position that this framework has in HunyuanCustom. LLaVA is used to generate image captions and assist in aligning visual content with text prompts, supporting the construction of a coherent training signal across modalities:
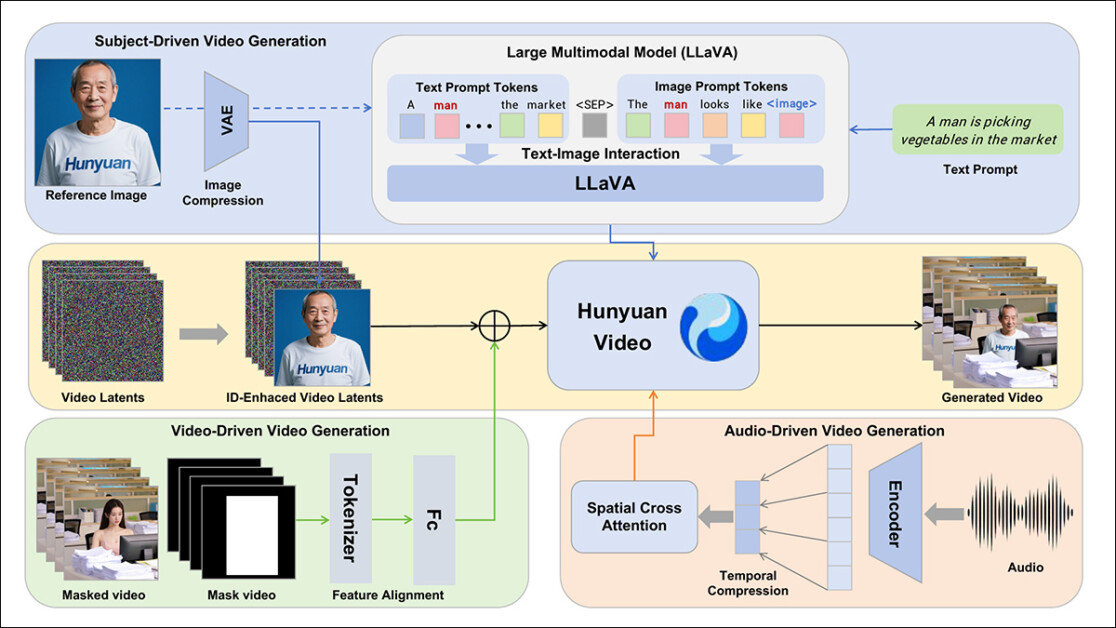
The HunyuanCustom framework supports identity-consistent video generation conditioned on text, image, audio, and video inputs.
By leveraging LLaVA’s vision-language alignment capabilities, the pipeline gains an additional layer of semantic consistency between visual elements and their textual descriptions – especially valuable in multi-subject or complex-scene scenarios.
Custom Video
To allow video generation based on a reference image and a prompt, the two modules centered around LLaVA were created, first adapting the input structure of HunyuanVideo so that it could accept an image along with text.
This involved formatting the prompt in a way that embeds the image directly or tags it with a short identity description. A separator token was used to stop the image embedding from overwhelming the prompt content.
Since LLaVA’s visual encoder tends to compress or discard fine-grained spatial details during the alignment of image and text features (particularly when translating a single reference image into a general semantic embedding), an identity enhancement module was incorporated. Since nearly all video latent diffusion models have some difficulty maintaining an identity without an LoRA, even in a five-second clip, the performance of this module in community testing may prove significant.
In any case, the reference image is then resized and encoded using the causal 3D-VAE from the original HunyuanVideo model, and its latent inserted into the video latent across the temporal axis, with a spatial offset applied to prevent the image from being directly reproduced in the output, while still guiding generation.
The model was trained using Flow Matching, with noise samples drawn from a logit-normal distribution – and the network was trained to recover the correct video from these noisy latents. LLaVA and the video generator were both fine-tuned together so that the image and prompt could guide the output more fluently and keep the subject identity consistent.
For multi-subject prompts, each image-text pair was embedded separately and assigned a distinct temporal position, allowing identities to be distinguished, and supporting the generation of scenes involving multiple interacting subjects.
Sound and Vision
HunyuanCustom conditions audio/speech generation using both user-input audio and a text prompt, allowing characters to speak within scenes that reflect the described setting.
To support this, an Identity-disentangled AudioNet module introduces audio features without disrupting the identity signals embedded from the reference image and prompt. These features are aligned with the compressed video timeline, divided into frame-level segments, and injected using a spatial cross-attention mechanism that keeps each frame isolated, preserving subject consistency and avoiding temporal interference.
A second temporal injection module provides finer control over timing and motion, working in tandem with AudioNet, mapping audio features to specific regions of the latent sequence, and using a Multi-Layer Perceptron (MLP) to convert them into token-wise motion offsets. This allows gestures and facial movement to follow the rhythm and emphasis of the spoken input with greater precision.
HunyuanCustom allows subjects in existing videos to be edited directly, replacing or inserting people or objects into a scene without needing to rebuild the entire clip from scratch. This makes it useful for tasks that involve altering appearance or motion in a targeted way.
Click to play. A further example from the supplementary site.
To facilitate efficient subject-replacement in existing videos, the new system avoids the resource-intensive approach of recent methods such as the currently-popular VACE, or those that merge entire video sequences together, favoring instead the compression of a reference video using the pretrained causal 3D-VAE – aligning it with the generation pipeline’s internal video latents, and then adding the two together. This keeps the process relatively lightweight, while still allowing external video content to guide the output.
A small neural network handles the alignment between the clean input video and the noisy latents used in generation. The system tests two ways of injecting this information: merging the two sets of features before compressing them again; and adding the features frame by frame. The second method works better, the authors found, and avoids quality loss while keeping the computational load unchanged.
Data and Tests
In tests, the metrics used were: the identity consistency module in ArcFace, which extracts facial embeddings from both the reference image and each frame of the generated video, and then calculates the average cosine similarity between them; subject similarity, via sending YOLO11x segments to Dino 2 for comparison; CLIP-B, text-video alignment, which measures similarity between the prompt and the generated video; CLIP-B again, to calculate similarity between each frame and both its neighboring frames and the first frame, as well as temporal consistency; and dynamic degree, as defined by VBench.
As indicated earlier, the baseline closed source competitors were Hailuo; Vidu 2.0; Kling (1.6); and Pika. The competing FOSS frameworks were VACE and SkyReels-A2.
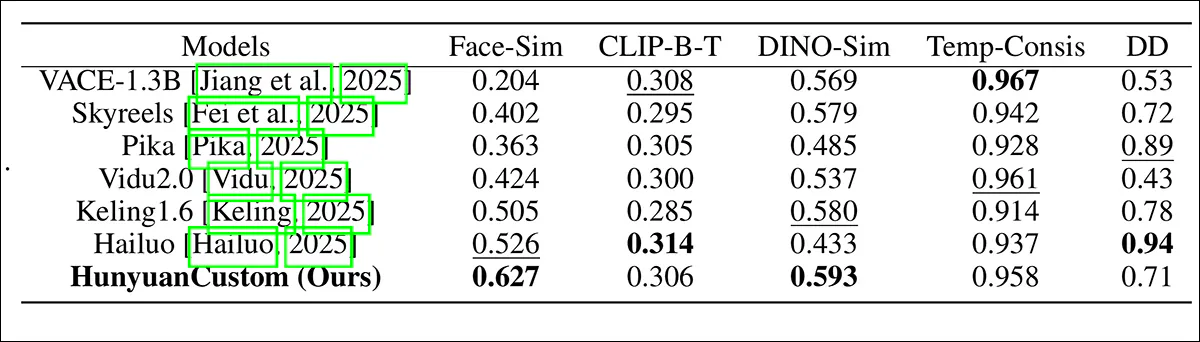
Model performance evaluation comparing HunyuanCustom with leading video customization methods across ID consistency (Face-Sim), subject similarity (DINO-Sim), text-video alignment (CLIP-B-T), temporal consistency (Temp-Consis), and motion intensity (DD). Optimal and sub-optimal results are shown in bold and underlined, respectively.
Of these results, the authors state:
‘Our [HunyuanCustom] achieves the best ID consistency and subject consistency. It also achieves comparable results in prompt following and temporal consistency. [Hailuo] has the best clip score because it can follow text instructions well with only ID consistency, sacrificing the consistency of non-human subjects (the worst DINO-Sim). In terms of Dynamic-degree, [Vidu] and [VACE] perform poorly, which may be due to the small size of the model.’
Though the project site is saturated with comparison videos (the layout of which seems to have been designed for website aesthetics rather than easy comparison), it does not currently feature a video equivalent of the static results crammed together in the PDF, in regard to the initial qualitative tests. Though I include it here, I encourage the reader to make a close examination of the videos at the project site, as they give a better impression of the outcomes:
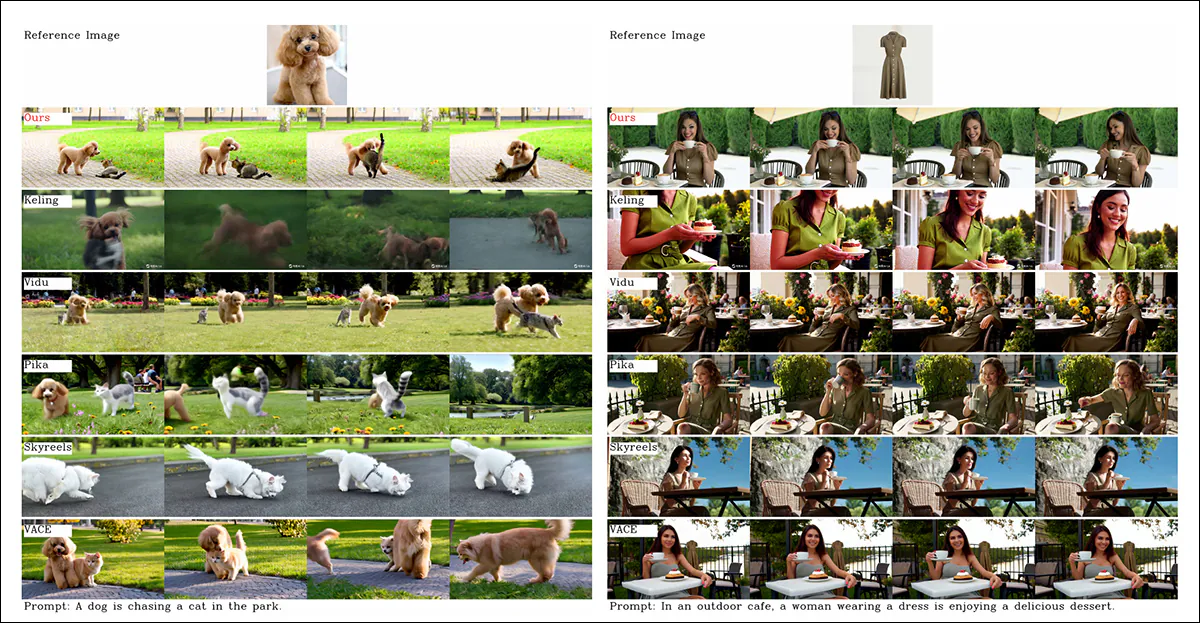
From the paper, a comparison on object-centered video customization. Though the viewer should (as always) refer to the source PDF for better resolution, the videos at the project site might be a more illuminating resource in this case.
The authors comment here:
‘It can be seen that [Vidu], [Skyreels A2] and our method achieve relatively good results in prompt alignment and subject consistency, but our video quality is better than Vidu and Skyreels, thanks to the good video generation performance of our base model, i.e., [Hunyuanvideo-13B].
‘Among commercial products, although [Kling] has a good video quality, the first frame of the video has a copy-paste [problem], and sometimes the subject moves too fast and [blurs], leading a poor viewing experience.’
The authors further comment that Pika performs poorly in terms of temporal consistency, introducing subtitle artifacts (effects from poor data curation, where text elements in video clips have been allowed to pollute the core concepts).
Hailuo maintains facial identity, they state, but fails to preserve full-body consistency. Among open-source methods, VACE, the researchers assert, is unable to maintain identity consistency, whereas they contend that HunyuanCustom produces videos with strong identity preservation, while retaining quality and diversity.
Next, tests were conducted for multi-subject video customization, against the same contenders. As in the previous example, the flattened PDF results are not print equivalents of videos available at the project site, but are unique among the results presented:
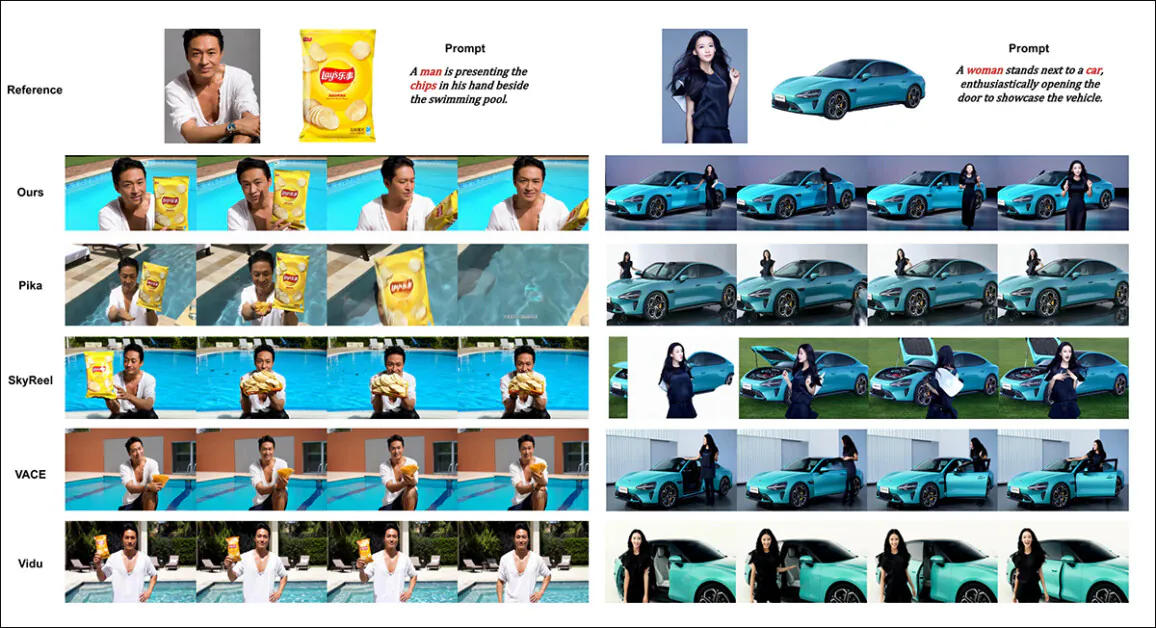
Comparisons using multi-subject video customizations. Please see PDF for better detail and resolution.
The paper states:
‘[Pika] can generate the specified subjects but exhibits instability in video frames, with instances of a man disappearing in one scenario and a woman failing to open a door as prompted. [Vidu] and [VACE] partially capture human identity but lose significant details of non-human objects, indicating a limitation in representing non-human subjects.
‘[SkyReels A2] experiences severe frame instability, with noticeable changes in chips and numerous artifacts in the right scenario.
‘In contrast, our HunyuanCustom effectively captures both human and non-human subject identities, generates videos that adhere to the given prompts, and maintains high visual quality and stability.’
A further experiment was ‘virtual human advertisement’, wherein the frameworks were tasked to integrate a product with a person:
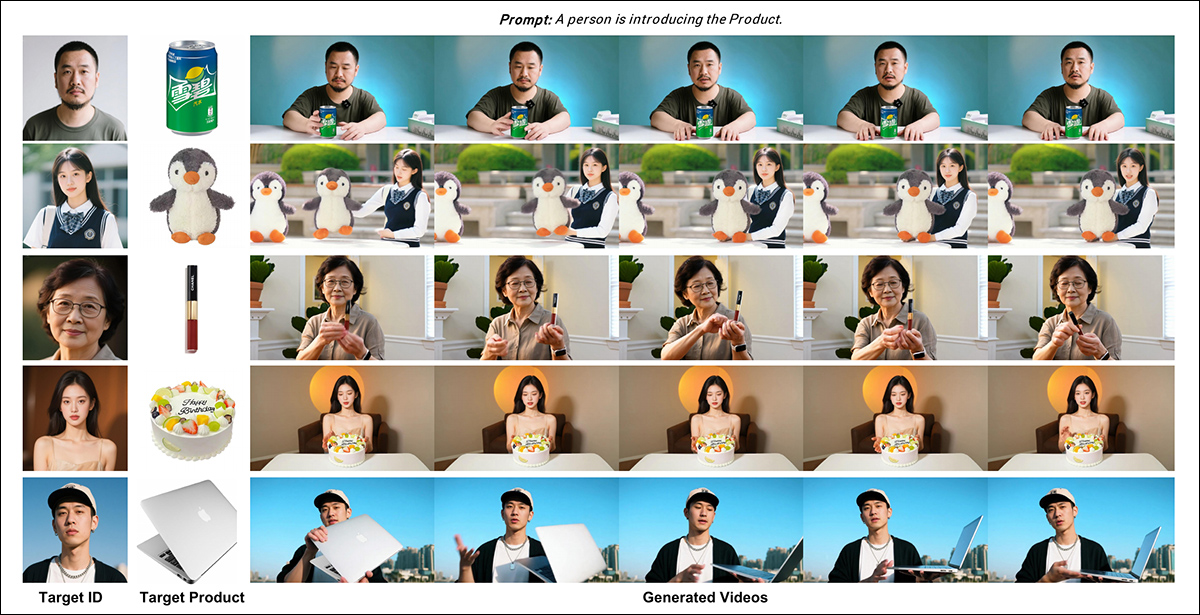
From the qualitative testing round, examples of neural ‘product placement’. Please see PDF for better detail and resolution.
For this round, the authors state:
‘The [results] demonstrate that HunyuanCustom effectively maintains the identity of the human while preserving the details of the target product, including the text on it.
‘Furthermore, the interaction between the human and the product appears natural, and the video adheres closely to the given prompt, highlighting the substantial potential of HunyuanCustom in generating advertisement videos.’
One area where video results would have been very useful was the qualitative round for audio-driven subject customization, where the character speaks the corresponding audio from a text-described scene and posture.
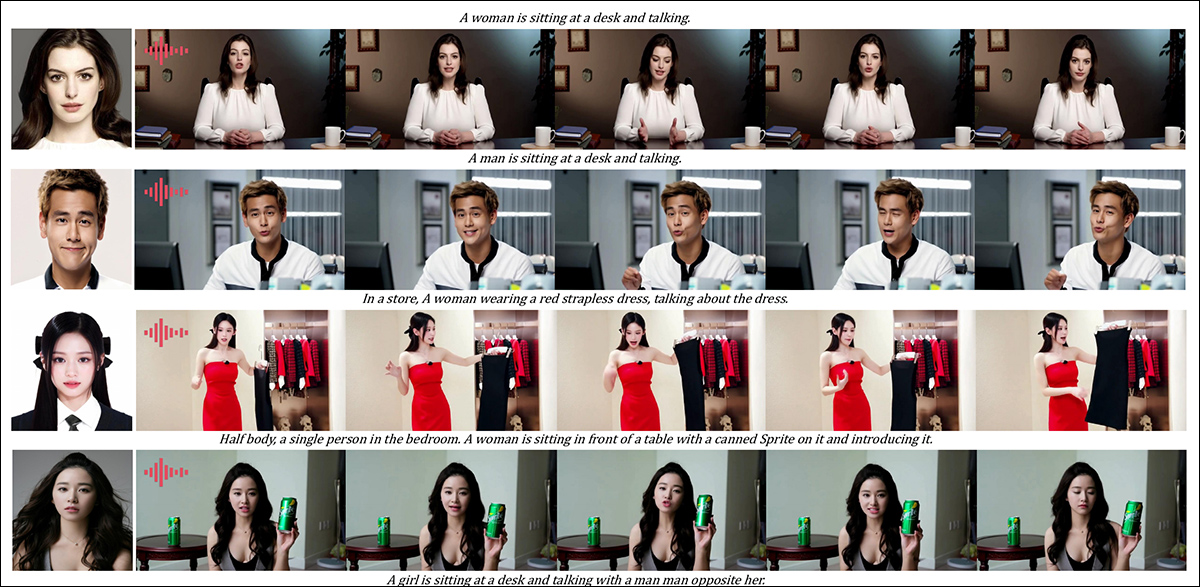
Partial results given for the audio round – though video results might have been preferable in this case. Only the top half of the PDF figure is reproduced here, as it is large and hard to accommodate in this article. Please refer to source PDF for better detail and resolution.
The authors assert:
‘Previous audio-driven human animation methods input a human image and an audio, where the human posture, attire, and environment remain consistent with the given image and cannot generate videos in other gesture and environment, which may [restrict] their application.
‘…[Our] HunyuanCustom enables audio-driven human customization, where the character speaks the corresponding audio in a text-described scene and posture, allowing for more flexible and controllable audio-driven human animation.’
Further tests (please see PDF for all details) included a round pitting the new system against VACE and Kling 1.6 for video subject replacement:
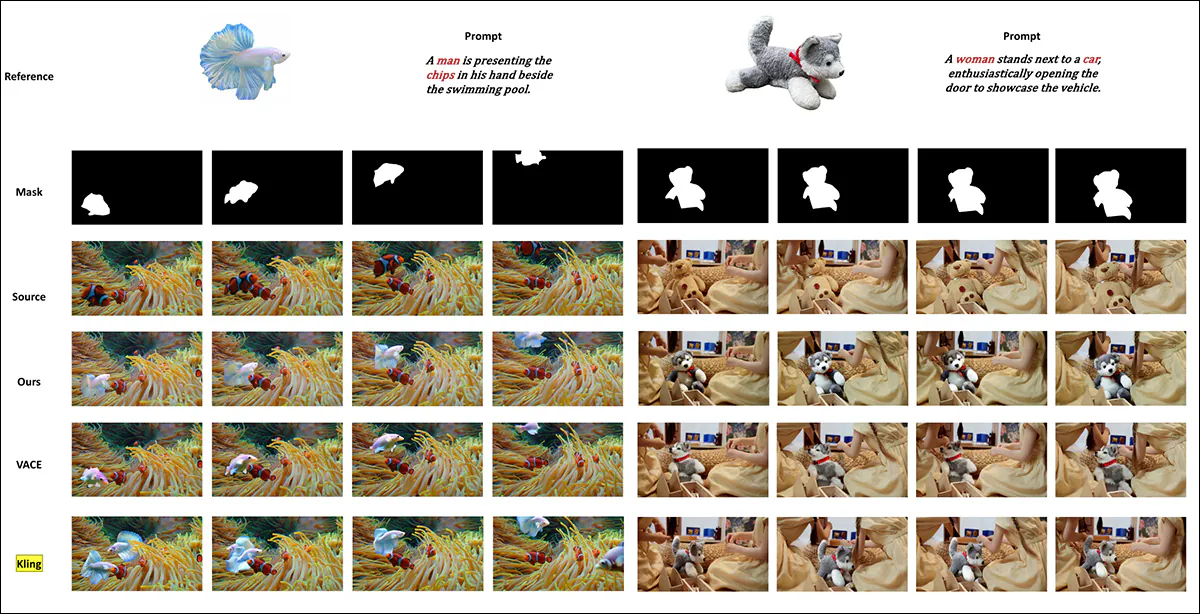
Testing subject replacement in video-to-video mode. Please refer to source PDF for better detail and resolution.
Of these, the last tests presented in the new paper, the researchers opine:
‘VACE suffers from boundary artifacts due to strict adherence to the input masks, resulting in unnatural subject shapes and disrupted motion continuity. [Kling], in contrast, exhibits a copy-paste effect, where subjects are directly overlaid onto the video, leading to poor integration with the background.
‘In comparison, HunyuanCustom effectively avoids boundary artifacts, achieves seamless integration with the video background, and maintains strong identity preservation—demonstrating its superior performance in video editing tasks.’
Conclusion
This is a fascinating release, not least because it addresses something that the ever-discontent hobbyist scene has been complaining about more lately – the lack of lip-sync, so that the increased realism capable in systems such as Hunyuan Video and Wan 2.1 might be given a new dimension of authenticity.
Though the layout of nearly all the comparative video examples at the project site makes it rather difficult to compare HunyuanCustom’s capabilities against prior contenders, it must be noted that very, very few projects in the video synthesis space have the courage to pit themselves in tests against Kling, the commercial video diffusion API which is always hovering at or near the top of the leader-boards; Tencent appears to have made headway against this incumbent in a rather impressive manner.
* The issue being that some of the videos are so wide, short, and high-resolution that they will not play in standard video players such as VLC or Windows Media Player, showing black screens.
First published Thursday, May 8, 2025
Credit: Source link