Collective intelligence improves the effectiveness of groups, organizations, and societies by utilizing distributed cognition and coordination, often facilitated by technologies such as online prediction markets and discussion forums. While LLMs like GPT-4 introduce crucial discussions around understanding, ethics, and the potential for artificial general intelligence, their effects on collective intelligence processes—such as civic engagement and interpersonal communication—are still largely unexamined yet increasingly relevant in today’s digital landscape.
The research examines how LLMs are reshaping collective intelligence, identifying both the advantages and challenges they introduce. By drawing on insights from multiple fields, the authors highlight the potential benefits and risks linked to LLMs, as well as important policy implications and research gaps. They stress the necessity for further exploration of how LLMs can affect our capacity for collective problem-solving. The study wraps up by identifying critical areas for attention among researchers, policymakers, and technology developers as they engage with this rapidly changing environment.
Collective intelligence (CI) refers to the capability of groups to act in ways that reflect intelligence greater than that of individuals working alone, particularly in areas such as idea generation, problem-solving, and decision-making. CI operates at various scales, from large markets where individual buyers and sellers interact to smaller teams coordinating efforts to overcome personal limitations. Key components fostering CI include diversity among individuals, individual competence suited to the task, and effective aggregation mechanisms that combine individual contributions into collective outcomes. Diversity, both demographic and functional, enhances problem-solving capabilities. In contrast, individual competence must align with the group’s knowledge level. Proper aggregation mechanisms, whether formal or informal, are crucial to facilitate meaningful interaction and minimize pitfalls like groupthink.
Recent technological advancements, particularly LLMs, offer new avenues for enhancing CI. These models, trained on extensive data from diverse sources, can facilitate collaboration by increasing accessibility and inclusion in online environments. LLMs can break down language barriers through translation, provide writing assistance, and summarize information, making it easier for participants to engage without becoming overwhelmed. Moreover, personal LLMs could represent individuals in discussions, streamlining deliberative processes. Overall, LLMs present significant opportunities for fostering larger, more diverse, and equitable online collaborations while posing challenges that need careful consideration.
Groups can enhance their ideation processes by integrating knowledge from diverse fields, often leading to innovative breakthroughs. LLMs present an opportunity to facilitate this process by mediating deliberative practices. They can help individuals engage in meaningful discussions by reducing cognitive load and providing structured support. For instance, LLMs can prompt participants to express their views more clearly or assist in organizing the conversation, thereby making deliberative processes more accessible and effective. Research shows that using LLMs in deliberation can increase participant satisfaction and foster a sense of trust and empathy.
However, the reliance on LLMs also poses risks to CI. The use of LLMs may discourage individual contributions to shared knowledge platforms, as people might prefer the efficiency of LLM-generated content over engaging with original sources. This reliance could lead to a homogenization of perspectives, diminishing functional diversity within groups. Additionally, LLMs can perpetuate illusions of consensus by amplifying commonly held beliefs while neglecting minority viewpoints, which can mislead individuals into thinking a consensus exists where it does not. To mitigate these challenges, promoting truly open LLMs, improving access to computational resources for diverse research, and implementing third-party oversight of LLM use are essential steps.
Check out the Paper. All credit for this research goes to the researchers of this project. Also, don’t forget to follow us on Twitter and join our Telegram Channel and LinkedIn Group. If you like our work, you will love our newsletter..
Don’t Forget to join our 50k+ ML SubReddit
⏩ ⏩ FREE AI WEBINAR: ‘SAM 2 for Video: How to Fine-tune On Your Data’ (Wed, Sep 25, 4:00 AM – 4:45 AM EST)
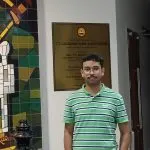
Sana Hassan, a consulting intern at Marktechpost and dual-degree student at IIT Madras, is passionate about applying technology and AI to address real-world challenges. With a keen interest in solving practical problems, he brings a fresh perspective to the intersection of AI and real-life solutions.
Credit: Source link